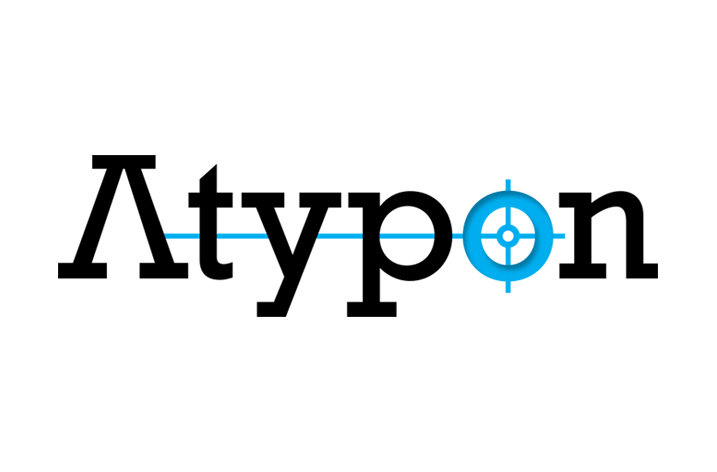
For the fifth year in a row, Atypon has placed in the widely respected International BioASQ Awards competition. Atypon’s ongoing research and development (R&D) into artificial intelligence technologies led to four awards for four different semantic technology categories in the 2017 BioASQ Challenge.
BioASQ organizes international contests in biomedical semantic indexing and question answering (QA) to help advance technologies that make it faster and easier for researchers to find the most relevant and actionable information from within the vast corpus of published biomedical research.
Atypon’s R&D in machine learning and natural language processing—two branches of artificial intelligence—contributed to their award-winning semantic technologies. The R&D team’s work in the areas of recommender systems and knowledge representation—specifically, entity recognition, entity extraction, semantic search, search augmentation, and publication knowledge graphs—is also expanding the search and discovery capabilities of Literatum, the company’s online publishing platform.
Entity recognition, a type of semantic enrichment, uses deep inference and reasoning to improve the accuracy, speed, and ease with which content can be automatically assessed and classified.
Entity extraction, another type of semantic enrichment, will be used to automatically add funding and grant information to enrich an article’s metadata, as well as to individuate compound figures and then match them with their captions and other associated content.
Semantic search, a type of search augmentation, will enable Literatum users to ask natural-language questions rather than search by keyword. And publication knowledge graphs that make use of knowledge representation technology will improve individualized content recommendations and predict trending research topics.
Previous AI-related innovations from Atypon’s R&D team are already featured in Literatum, including an auto-tagger that helps personalize Literatum search results and auto-populate topic-specific web pages with relevant content, search augmentation algorithms that rank and organize results according to each user’s interests, and automated topic modeling that analyzes a set of documents, determines their subject matter, and instantly assigns tags that characterize each topic.
All of these Literatum features make valuable content and information easier to find and monetize, and create a more productive, convenient research experience.
Atypon’s BioASQ team was joined by professors from Aristotle University in Thessaloniki, Greece, a research institution that Atypon works closely with on projects combining machine learning, natural language processing, and other applied software technologies.